DAISY SUITE
The transformations that Information Systems organizations constantly face, as a reflection of frequent and significant changes in the enterprise structure, create a need for more and more new testing environments that are comprehensive in terms of accurate test data.
Often, to ensure the completeness of any required functional tests, clients resort to the creation of data bases mirroring actual production data, causing considerable consumption of resources.
An exhaustive “Subsetting” functionally and detailed “Data Masking” must adhere to the full requirements of the confidentiality of personal information. This is also the optimal solution in accordance with increasingly strict and monitored national and international standards with regard to privacy, in order to prevent the recognition of personal information and other sensitive data from internal and external staff.
THE DAISY SUITE SOLUTION
Daisy Suite is the cross-platform solution that fully responds to the needs of subsetting and masking of client data, guaranteeing throughout all
phases of preparation of various test databases, the correct technological support that would facilitate and accelerate the definition and implementation of processes. A truly automated solution such as Daisy is vastly superior to the manual skills that are typically employed in a traditional context for in-house creation of test data.
Daisy provides an automated, standard approach, based on knowledge of the data and potentially applicable to various sectors involved: Application Development, Data and DB Administration, Operations, QA, etc..
The web interface and intuitive navigation will facilitate the accessibility for users that, building interactively reduction processes and data masking rules, are able to create their own subsetting and masking model, ready to be implemented and repeated according to the specific requirements.
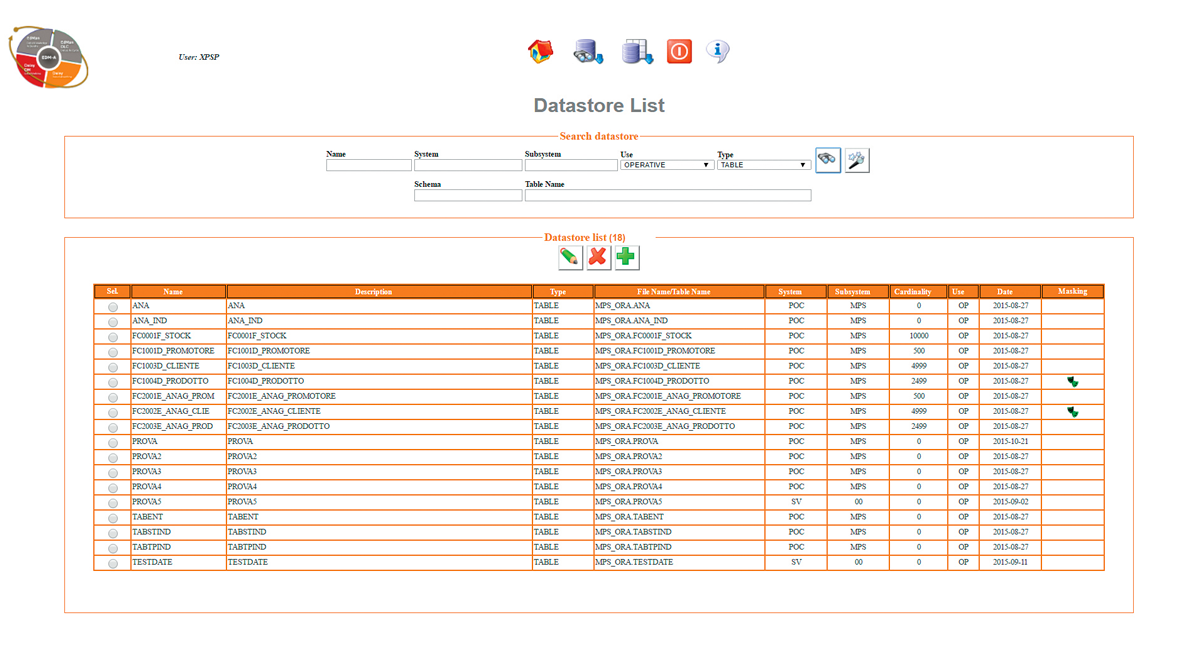
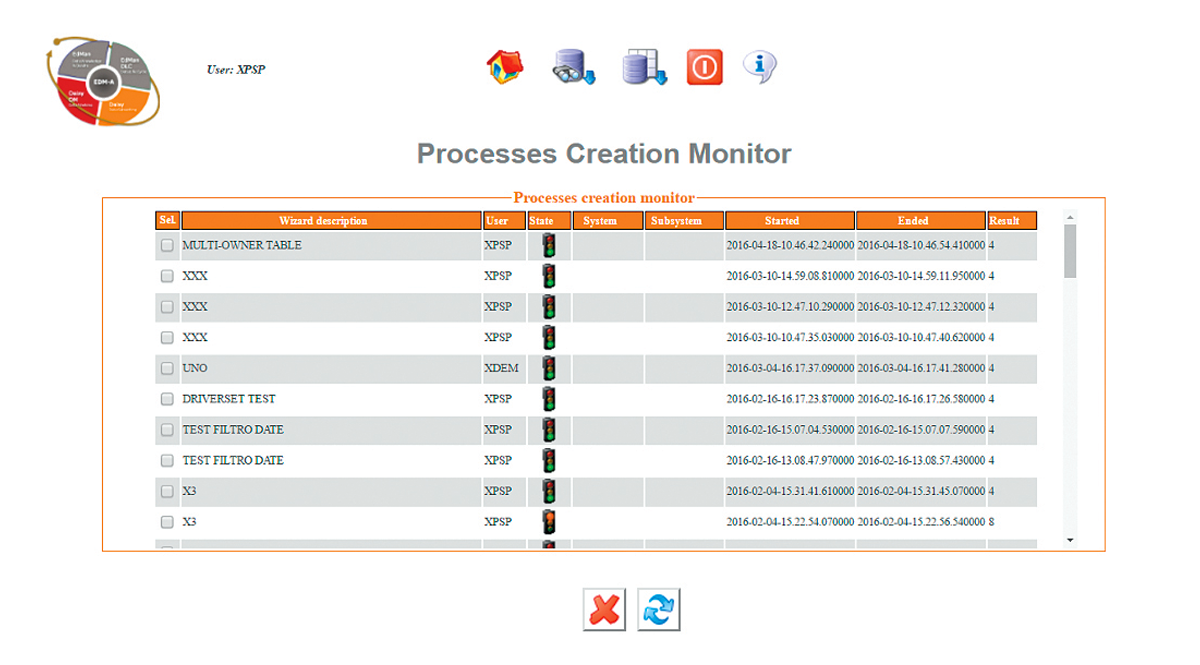
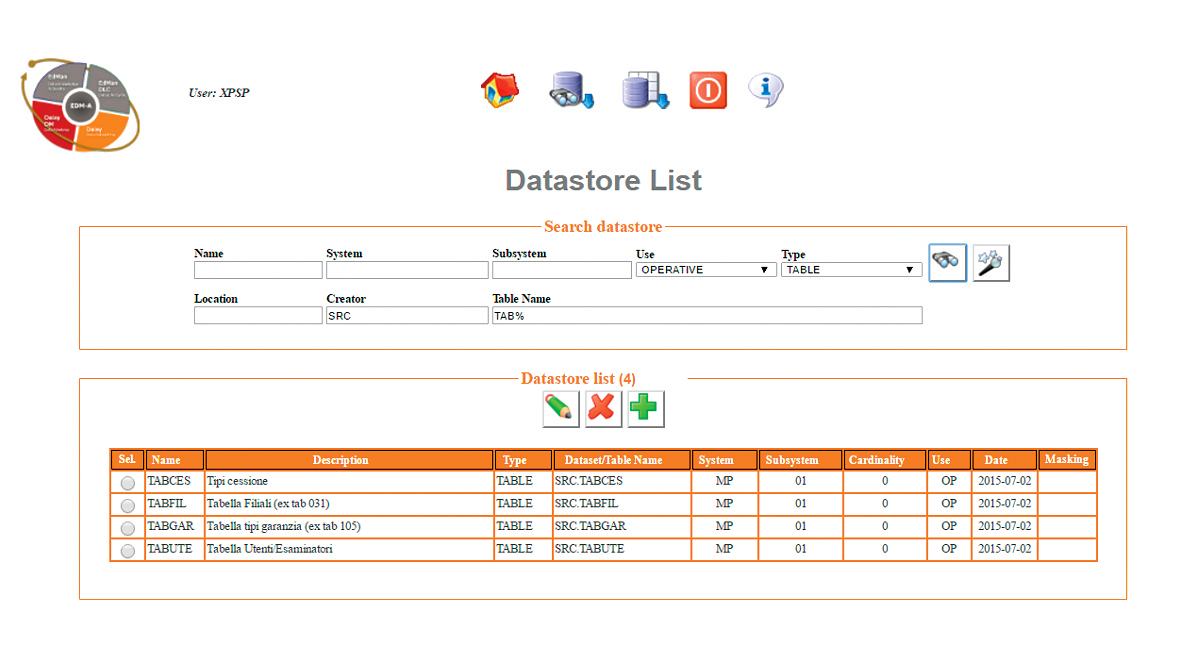
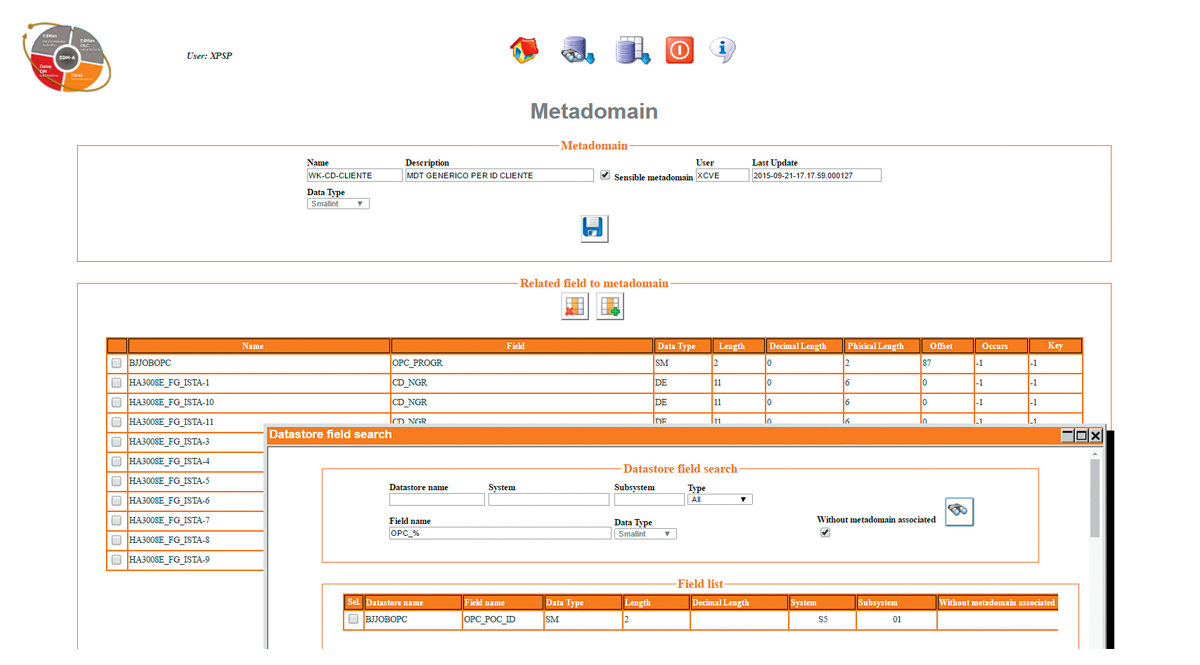
OBJECTIVES AND FUNCTIONS OF DAISY SUITE
The central tool repository contains the descriptive elements of the archives (relational DB2/Oracle/ SQLServer – File: text fixed records, CSV, VSAM, Sequential, …), for process definition elements that act on them, in a highly detailed coded format, which is usable in the various phases of any subsetting/masking project:
- The administrative phase, which allows the user to “tailor” Daisy, utilizing the repository, according to a principle of minimization of manual intervention. The numerous “wizards” available allow the user to automate repetitive tasks such as discovery of the definition of archives and their relationships or the generation of large numbers of replication processes or extraction. At this stage they also define the masking rules.
- The creation phase, which provides for the customization and automatic generation of batch scripts oriented to the execution platform (operating system and DBMS).
- The implementation phase, in which the generated batch are carried out by the “process engines” specific for the platform, according to the predetermined intervals or on user request.
Subsetting:
Daisy provides several strategies for reducing data in respect to completeness and consistency required objectives:
- Selection based on generalized filters made of metadata using specific values or lists of values;
- Selection based on “Driver Tables”, and led by “keys” extracted from higher level subsetting processes;
- Selection based on user-defined complex Administrator filters;
- Total replication (for particular classes of archives, eg.: domain tables).
Masking:
Daisy has its own powerful language for the definition of the masking rules, and is characterized by:
- Native masking functions for standard type fields (Tax Code / Credit Card Number / Unique Codes / Dates / Zip Codes / …)
- Lookup tables for customizing the non-key fields masking text (names / surnames / corporate names / Locations / addresses …)
- Masking contextual data extraction,for a more complete respect for privacy requirements
- Required data discovery for automatic recognition of Data Domains.Denominazioni societarie /Località /Indirizzi…)
The subject of data masking meets important requirements of the transformation:
- Repeatability: the same transformation at any time reproduces the same results on equal terms
- Consistency: maintain the functional relationship between data, such as the relationship between Surname, Name, Date of birth and tax code
- Likelihood: the masked figures are as close to realistic as the original
- Homogeneity cross-platform: data from different platforms can be masked in a homogeneous and coherent manner
- Irreversibility of masking: it is impossible to go
back to the original data.
BENEFITS
- Unique access point across a multi- platform management environment (same approach for z/OS and LUW)
- Simplified management of classic subsetting procedures
- Simplified management of classic subsetting procedures
- Highly flexible and modular approach to the issues
- No need to write custom code
- Little work needed for the maintenance process.